Research Data Management
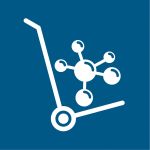
Knowing how to organize and manage research data is one of the most important prerequisites for ensuring the quality, security, durability, and reproducibility of research data, both during a research project and in long-term. Data Stewards advise, support individually and in groups through workshops and events around open research data and open science. If you have any questions about research data management and data management plans, please contact us via E-Mail: researchdata@unibe.ch
Support
New Walk-in consultation for all researchers
Data Stewards are offering a new “Walk-in” consultation for all researchers from the University of Bern and the Insel University Hospital every Monday from 2 pm to 4 pm during semester. Whether you look for a convenient way to organize data in your project folder, whether you need help developing a data management plan (DMP) for your project or whether you don’t know how to publish your data – just drop in at our office in Hochschulstrasse 6, room 354 and we’ll do what we can to help you!
Your personal contact for research data management
Our research data management support is now even more focused on the specific issues of academic domains (groups of disciplines). You will also receive additional support for overarching issues such as handling sensitive data and data science. Data stewards are now available to you as contact persons for each domain and the overarching issues. They will support you in close cooperation with other support and infrastructure facilities of the University of Bern in the fulfillment of data-related requirements of the funding institutions (e.g. SNSF, EU, NIH) and generally in the implementation of good practices in research data management.
Do you have any questions? Do you need support with research data management? Write to researchdata@unibe.ch – your data steward will get back to you promptly!
Data Management Plan (DMP)
For all SNSF calls a data management plan (DMP) is requested for approved grants only (SNSF). Researchers still have to consider data management when planning their projects and preparing applications, including budgeting Read more
We review your DMP for the Swiss National Science Foundation SNSF or other funding organizations within 2-3 working days. If possible, please also send us your project plan so that we can give you customized feedback. You can find an introduction to writing a DMP for the SNSF in five short videos on YouTube. You can also take a look at our collection of DMP examples and submit your DMP for review here. This service is free of charge.
Research Data Repository of the University of Bern
On BORIS Portal you can publish your research data in open or restricted access according to the FAIR Data principles and link it to information about your research project. A factsheet with the most important information and a checklist for publishing datasets can be found here.
Research Data Management Guideline
With this guideline on Research Data Management (RDM), the Data Stewards at the Open Science Team in the University Library of Bern aim to support researchers from all faculties at the University of Bern and Insel Hospital in research data management throughout the project life cycle. This includes dealing with ethical data collection, data reuse, data generation and data processing, data documentation, and preparing data and metadata, as well as code, and software for publication.
Bern Medical Research Landscape
The DLF Insel and the Open Science Team jointly developed the Bern Medical Research Landscape to help researchers navigate the various offers of support and services at UniBE and the Insel Hospital.
DMP
What is research data?
Research data is "data collected or produced (e.g. measurements, questionnaires or source materials) in the course of scholarly activity which is used for the purposes of academic research (e.g. digital copies) or which document research findings [...]". forschungsdaten.info
Some examples of research data
- Sources: Texts, images, sound recordings, films/videos
- Observations: Real-time data, examination data
- Experiments: Laboratory values, spectrograms
- Simulations: Simulation measurements, model measurements
- References: Collection of already published datasets
- Methodological methods such as questionnaires, software or simulations
What is a data management plan?
A data management plan (DMP) forms the basis of good research data management. The DMP describes the life cycle of research data and is intended for long-term use. It describes how the data is to be produced, collected, documented, published and archived during a project.
An integral part of a DMP is the description of the research data in accordance with the FAIR principles. Among other things, a DMP should include information about the following:
Data collection and documentation
Ethical, legal and security issues
Data storage and preservation
Data exchange and reuse
The DMP is submitted along with the project proposal (SNF) or shortly after the project has commenced (H2020), and it should be updated and extended at regular intervals. As it describes discipline-specific practices and standards, the content may differ from project to project.
Funding requirements
The Open Science Research Data Management Team will be happy to support you by advising how to comply with funding agencies' requirements in Open Research Data for the European Commission (e.g., Horizon Europe) and other national (SNSF) and international projects. We offer individual support and training sessions as well as workshops based on your needs for you and your team on request. Please contact us via openscience@unibe.ch.
Swiss National Science Foundation (SNSF)
The DMP is an integral part of the research application. The application cannot be submitted until the DMP has been completed. The DMP must be written in the same language as the research plan. The SNSF contributes up to 10’000 CHF to the costs of making research data accessible, under the condition that the repositories used for data sharing meet certain requirements (AR 2.13). The application for this additional funding must be taken into account when submitting the application. For more information, see the SNSF guidelines for researchers.
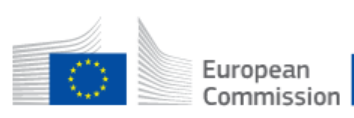
You must submit a DMP no later than six months after the start of the project to the EU’s project management portal. A template with guidance can be downloaded here.
All projects are part of the "Open Data Pilot", i.e. researchers must make research data underlying publications openly accessible. Exceptions for ethical, legal, contractual, copyright, and similar reasons are possible ("opt-out"), but they must be justified to the EU.
The European Research Council (ERC) requirements differ from the general Horizon2020 requirements only in details. This page gives a good overview and links to further resources and the ERC DMP form.
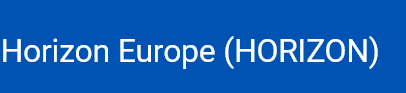
Information as of June 2022
Regarding participation in the Horizon Europe framework, Switzerland is considered as non-associated third country until further notice (more information). Substitute funding measures for Swiss researchers are mainly provided by the Swiss National Science Foundation (SNSF) and the State Secretariat for Education, Research and Innovation (SERI). More information on the Grants Office website.
For these replacement funding measures, guidelines for open access publishing and research data management apply as follows:
For the replacement measures by the SNSF, the SNSF Open Science requirements apply (see above and here).
For the substitute measures by SERI, the specifications of the European Union apply (see here). They stipulate that:
- Data must be managed according to the FAIR data principles. FAIR stands for Findable, Accessible, Interoperable, Reusable.
- A Data Management Plan (DMP) must be written, typically submitted six months after the start of the project, and updated at least at the end of the project period. It is recommended to use the official template.
- Research data must be shared openly on a repository if there are no legal, copyright, ethical, contractual or similar clauses. Unlike under Horizon2020, the EU requires a mandatory CC0 or CC BY license (or equivalents).
If you are unsure what these requirements mean for your project, please contact us at openscience@unibe.ch.
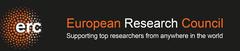

National Institutes of Health (NIH)
Effective January 25, 2023, the following guidelines apply to NIH-funded projects:
Researchers must develop and submit a data management plan together with the project application, and update it regularly. The NIH will review the DMP. They may also review implementation during the life of the project. Failure to comply may affect the success chances of future applications.
In addition, researchers must share research data as soon as possible, at the latest at the time of a related publication appears or at the end of the project (whichever comes first). Legal and ethical considerations must be taken into account.
Templates and examples
Please be aware that the funding agency can change the template. Please contact the research data management team via openscience@unibe.ch for details and further information.
Templates
- Data Management Plan – mySNF template
- DLCM DMP templates
- ERC-DMP template
- DMP EU Horizon 2020
- NIH Data Management and sharing. A preview of this format page is available now, with a final fillable format version available by Fall 2022.
Beispiele
- DMP-Beispielsammlung DCC (e.g., Horizon 2020)
- DLCM SNSF DMP template and guideline (PDF, 637KB)
- DMP examples SNF
- NIH DMP
- NIH Examples of Data Sharing Plans
DMP examples SNSF
DMP videos and slides
How to write a successful research data management plan (DMP)? The Open Science Team will be happy to guide you through video modules available via YouTube.
Documentation & Metadata
File organization
To avoid errors, mix-ups and long search times in future, it is worth investing some time in creating a systematically organized file and folder structure already at the start of a project. This is especially important if you are collaborating with other research groups. Everyone involved in a project should agree to a scheme and stick to it. It is advisable to record the organizational and naming scheme in a document which you subsequently deposit with the published data as an accompanying document.
- Group related files in folders (e.g. for measurements, methods or project phases)
- Use clear, unique folder names
- Use a hierarchical folder structure (N.B.: too many nested levels results in long and complicated filepaths)
- Keep active and completed work in separate folders and delete any temporary files that are no longer required.
File names
Make sure you use file names that are unique and are also meaningful for people who are not involved in the project. General elements that can form part of a name:
- Creation date (YYYY-MM-DD)
- Project reference/name
- Description of the content
- Name of creator (initials or whole name)
- Name of research team/department
- Version number
To avoid operating system constraints, use the following character/naming conventions:
- Short names
- No special characters (: & * % $ £ ] { ! @)
- Use underscores _ rather than blank spaces or dots
- Include a file suffix wherever possible (.txt, .xls, etc.)
- Do not rely on uppercase/lowercase distinctions
File formats
The careful choice of a file format can ensure that files can still be used after many years and consequently greatly facilitate reuse of the research data. When choosing a suitable format, various factors should be taken into consideration:
- Future-proofing: how many software products can read the data format?
- Open access to documentation
- No legal constraints (patents)
- No technical constraints (encryption, DRM)
- Established in community
The file formats for research data can vary widely depending on the discipline in question. The following file formats are recommended:
- Images: TIFF, TIF
- Documents: TXT, ASC, PDF/A
- Tabular data: CSV
- Audio files: WAV
- Databases: SQL, XML
- Structured data: XML, JSON, YAML
Further information about which file formats are recommended for long-term preservation can be found at here.
Version control
It is essential to use version control, especially for datasets that change over the course of a project. Individual datasets should be named sequentially and the names should include the save date (YYYY-MM-DD) along with the version number. The final version should be indicated as such. Maintaining a version table in which all changes and new names are recorded can help keep track of the datasets.
Especially when working with a number of different people, it may be advisable to regularly save a milestone version of the file which then must not be changed or deleted.
To summarize, forschungsdaten.info recommends:
- Use sequential numbering
- Include the date and version number in the name
- Use a version control table
- Specify who is responsible for providing the final files
- Use version control software for large data volumes
- Save milestone versions
Further information and best practices
- Wilson, G. et al. (2017): Good enough practices in scientific computing. PLoS Comput Biol 13(6): e1005510 https://doi.org/10.1371/journal.pcbi.1005510
- Free version control software
Data backup
We recommend you back up your data using the university's IT system as it collects the data campus-wide and redundantly backs it up to two state-of-the-art tape libraries.
Click here for more information: Campus Backup/Archive (access only via campus network)
You should always adopt the 3-2-1 backup strategy:
- 3 copies of the data (1 original + 2 backups)
- Stored on 2 different types of media (external hard drives, USB sticks, SD cards, CDs, DVDs, Cloud)
- 1 copy off-site
Backup should be automated to run at regular intervals. Check that the backup was successful and that the data can be retrieved again if necessary.
Documentation
Comprehensive documentation is essential to enable correct interpretation and reuse of the data at a later date. Among other things, the documentation should include details about the time and place the data was collected, the methods, tools, software and statistics models used, as well as information about the parameters chosen and any missing values, along with nomenclature and acronyms. This information can be added complementary to your dataset, e.g., in the form of supplementary documentation in a ReadMe file.
Further information on data documentation can be found here and on ReadMe files here.
Metadata
Metadata is information about data which is created in a structured and machine-readable form. The metadata helps other researchers find and reuse data. Depending on the particular discipline, there are various commonly used metadata standards and tools that can be used to describe datasets in different domains.
The repository of the University of Bern (BORIS Publications) (BORIS) uses the Dublin Core metadata element set. This metadata is automatically generated by filling in a form when depositing a dataset in the repository.
Data quality and metadata standards. The presentation link is under the BORIS Publications.Sharing & Reuse
Selecting data
The decision about what data for a project should be archived and for how long depends on the academic value of the data as well as on legal, regulatory and financial factors.
As a minimum, however, all the data on which a publication is based must be stored and the corresponding metadata must be published online.
The Digital Curation Centre (DCC) and forschungsdaten.info list five steps for deciding what data to keep.
Finding a repository
Whenever possible, data should be deposited in subject-specific repositories. These are geared to the needs of the subject area, are familiar with specific data formats and often also offer subject-specific metadata.
Which data repositories can be used? A comprehensive list of data repositories is provided by the SNSF (the SNSF list is not exhaustive) and Scientific Data.
The best starting point for your search for a suitable repository is Research Data Repositories (re3data.org).
An institutional data repository (BORIS Portal Research Data, Research Project, Research Funding) has been officially launched. BORIS Portal allows you to archive and manage research data, to determine access options and manage rights, as well as to link project and researchers’ profiles, to make it accessible and clearly identifiable. Login to BORIS Portal research data, projects and fundings via your campus account.
Sharing
Sharing figure
Figshare - store, share and discover research.
Sharing methods
protocols.io A secure platform for the development and exchange of reproducible methods.
Choose a license
The Open Science Team at the University Library of Bern recommends licensing research data under the Creative Commons Public Domain Dedication (CC 0) or the newest version of the Creative Commons Attribution International Public License CC BY licenses to allow maximal reusability. If data cannot be openly published due to ethical or legal reasons, metadata and supplementary material can be published under CC0 to fulfil funders' agencies' requirements.
The Swiss National Science Foundation (SNSF) allows researchers to choose the best suitable license for the data based on the principle of reusability (e.g., SNSF Policy on Open Research Data).
The European Commission (EC) requires the latest available version of the CC BY or CC 0 or a licence with equivalent rights, following the principle “as open as possible as closed as necessary”. For details, please visit Horizon Europe Model Grant Agreement from 15. December 2021, v.1.1, p. 96 and the EU's open science policy (link).
Persistent identifiers
As part of the FAIR principles, funding bodies require a unique identifier to be assigned to the published data. When depositing your data in BORIS, a Digital Object Identifier (DOI) is assigned to each dataset. Click here for further information.
Publishing data
Research data generated and collected during a project can often be useful beyond its original purpose. It is therefore worthwhile making the data obtained publicly accessible. For this purpose it is important to ensure that your data is assigned persistent identifiers, good metadata is generated and sufficient documentation is provided to enable the data to be reused.
There are currently three ways of publishing research data.
Publication in a repository
Research data can be published in a disciplinary or a general repository. If possible, it is preferable to publish data in a disciplinary repository rather than in a generic one. Further information about selecting a suitable repository can be found in Finding a repository.
Publication in a data journal
Data papers published in data journals are documents that facilitate the dissemination and reuse of published data. These publications contain all information about data collection, methods, licenses and access rights along with information about potential reuse opportunities. The data itself is usually deposited in a repository.
The website of the Humboldt University of Berlin has a list of data journals.
Publication as a supplement to an article
Data can also be published as additional information for an article in a periodical. This is usually the data on which the publication is based which enables the findings to be understood. The data may either be deposited directly on the periodical's platform or in an external data repository.
Citing data
When citing data it is advisable to use either the standards applicable to the research field in question or the form suggested by the repository in which the dataset was deposited. If there are no particular standards or recommendations, Datacite recommends providing the following details as a minimum:
- Author
- Year of publication (of the dataset)
- Title
- Edition or version (optional)
- Publisher (for data this is usually the archive in which the data is stored)
- Resource type (optional)
- Persistent identifier (as a permanent linkable URL)
Open Source Software
Information and action guide for publishing open source software.
Data Protection
Ethics in Research Data Management
Data ethics, protection and security challenges as well as research integrity issues are closely related to the Open Science strategy, which aims to promote transparency and reusability of research data and knowledge transfer.
The Data Stewards in the Open Science Team support researchers at the University of Bern and Bern University Hospital by offering regular training courses and upon request. We support you by advising on how to collect, reuse, process, store, share and publish research data according to the best research practice, legal regulations, funding agencies requirements and ethical standards in Open Science.
Data Protection
Data security IT-Department, University of Bern (German version only, Pdf)
- Legal service office of the University of Bern (data protection, legal questions) E-Mail
- Unitectra supports researchers in the commercialization of research results into new products and services (patents, licenses), in the negotiation of research agreements as well as in the creation of a spin-off company E-Mail
Data protection and research in general: EDÖB (addresses federal bodies and private persons, here for general information in German, French and Italian only). Researchers at the University of Bern must normally comply with the Cantonal Data Protection Act).
European General Data Protection Regulation (GDPR). Introductory Ordinance to the EU Data Protection Directive 2016/680 on the protection of personal data (Introductory Ordinance to the EU Data Protection Directive) of the Canton of Bern.
The data protection laws of some countries are recognized as equivalent to those of Switzerland (Transborder data flows).
Personal data
Personal data is information about specific or identifiable natural or legal persons. The Data Protection Act of the Canton of Bern (KDSG) serves to protect individuals from improper data processing by authorities.
If you collect, analyze or otherwise process personal data in your research project with the help of IT applications (e.g. self-programmed apps, but also applications such as Qualtrics or RedCap), it may be necessary to draw up a concept for handling information security and data protection (ISDP).
Such a concept serves to clarify the requirements and existing or to be developed technical solutions in this area, as well as, if necessary, as the basis for control by the data protection authorities of the canton. The legal basis for this is the data protection legislation of the Canton of Bern, in particular, the Data Protection Act (KDSG), Art. 17a. The "Directives on Data Protection in the IT Sector of the University of Bern" must be followed (Link).
For information and advice on legal matters, please contact the Legal Services of the University of Bern, and for IT security matters and for filling out the relevant forms, please contact the IT Services.
Health-related data
Research projects involving humans that fall under the Human Research Act (HRA) must be reviewed by ethics committees (e.g. animal experiment ethics SAMS or Cantonal Ethics Committee (CEC)). Please note that such a review may take approximately 60-90 days. For more information on the procedure, process and management of such projects, please visit the portal on human research in Switzerland Kofam.
Research projects that do not fall under the Human Research Act can be approved by the Ethics Committees of the University of Bern Phil.-hum., Phil.-hist., WISO und Animal Welfare Office UniBE.
For information on research that falls under the Human Research Act, please refer to the "Ethics – Research with humans" tab. Information on research involving animals can be found under the "Ethics – Research with animals" tab. Information on working with genetic resources or their associated traditional knowledge can be found under the tab "Ethics – Research with genetic resources". More information can be found under Research Compliance and Good Research Practice.
Read more about ethics via forschungsdaten.info
Courses
The Data Stewards at the Open Science team support researchers at the University of Bern and Insel Hospital by offering training courses on regular and upon request.
Training and workshops in research data management aim to support researchers at the University of Bern and Insel to manage research data throughout the whole research data lifecycle from initiation, planning, and the start of the project until the end of the project. Moreover, many funding agencies, such as the Swiss National Science Foundation (SNSF) and the European Commission (e.g., H2020/Horizon Europe), require grant applicants to develop a data management plan (DMP) and demonstrate experience in data sharing and Open Science, Open Research Data. To learn more please register to our training courses and workshops, which are free of charge under the webpage here
Data Stewards
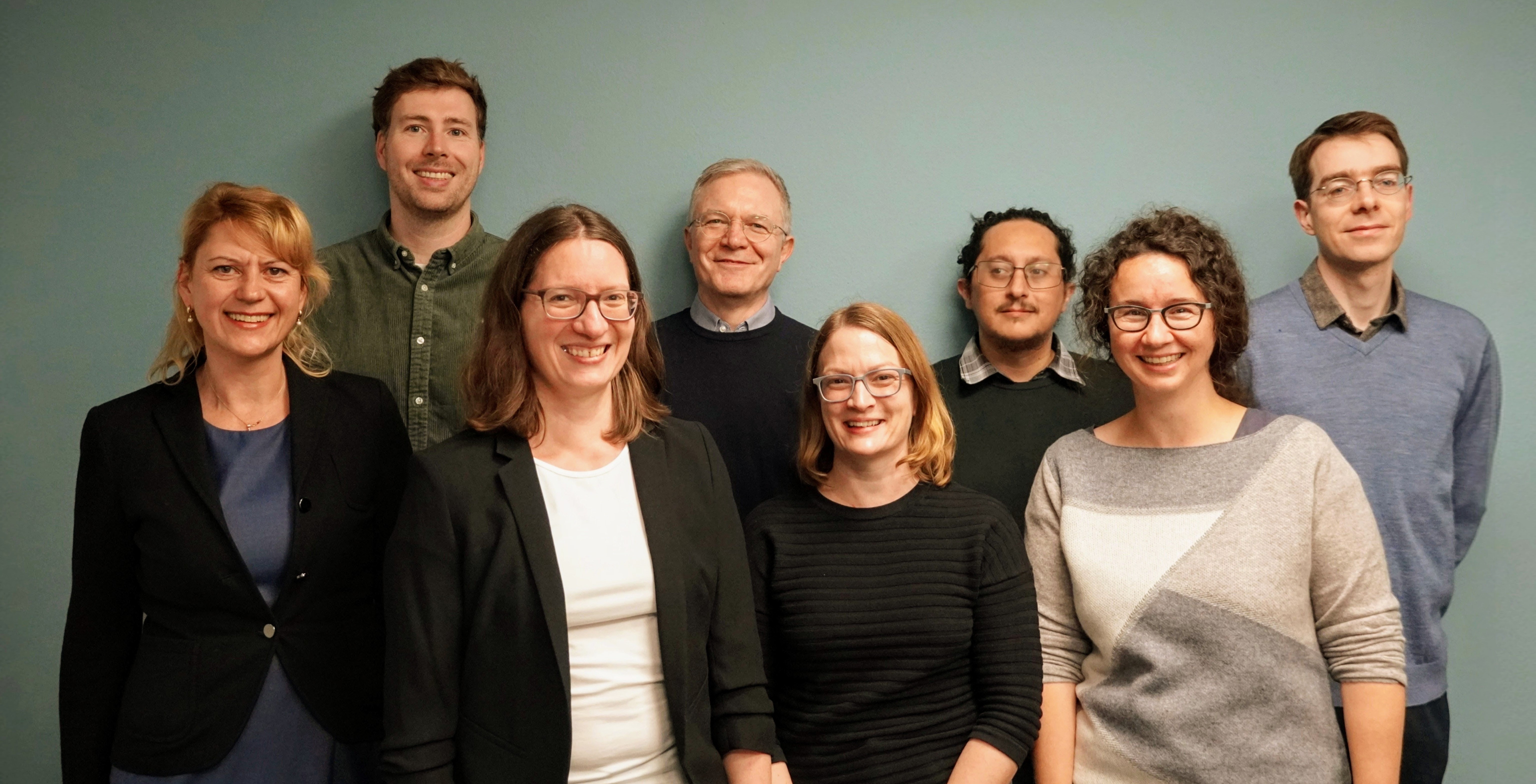
The Data Stewards support researchers at the University of Bern in all aspects of research data management (see Figure 1), from the planning phase to data storage and the publication of research data.
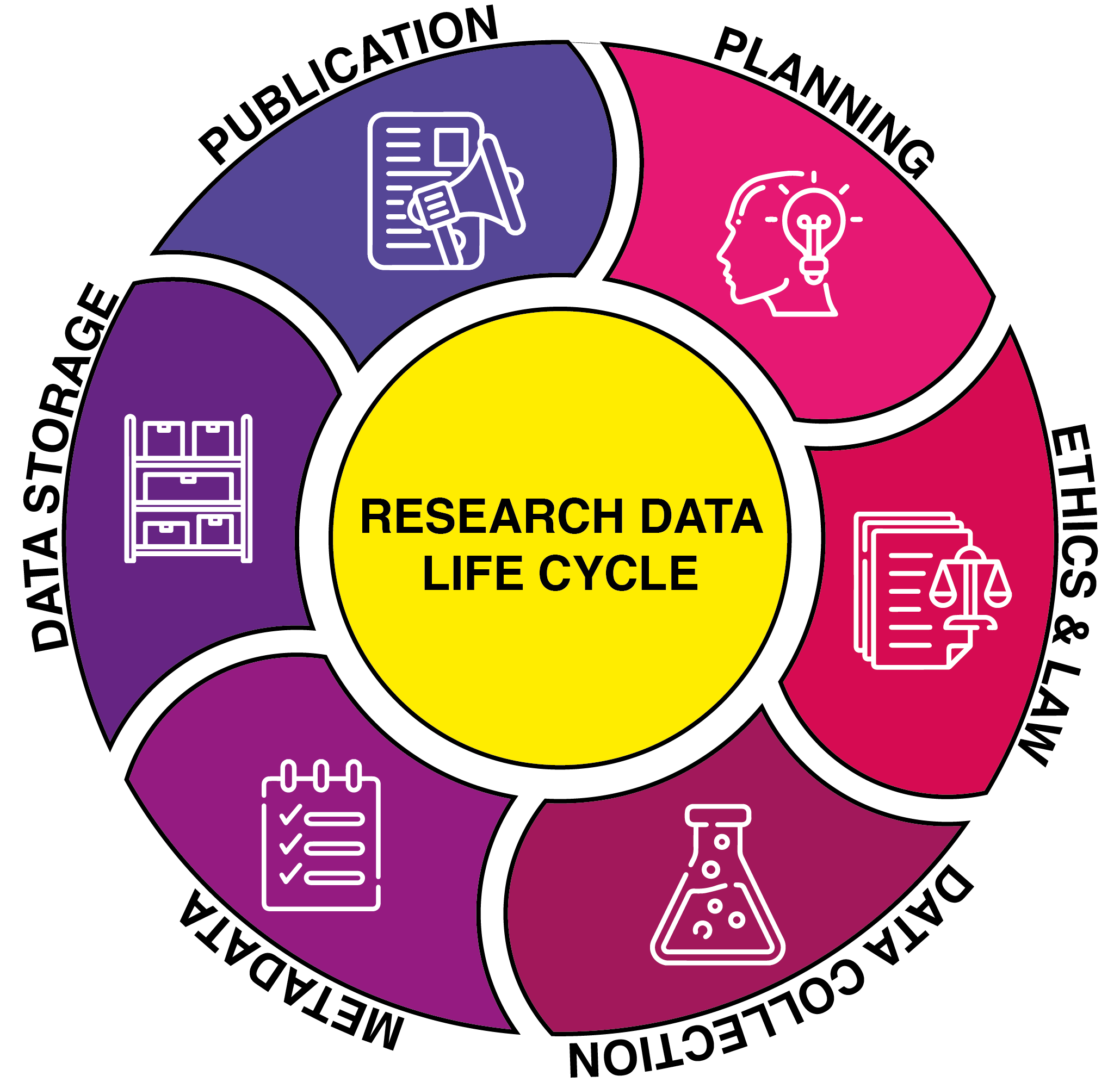
As the link between infrastructure and research, the Data Stewards provide domain-specific support for researchers and research units at the University of Bern. At the University of Bern, five domains and one cross-domain are covered by seven data stewards (see Figure 2):
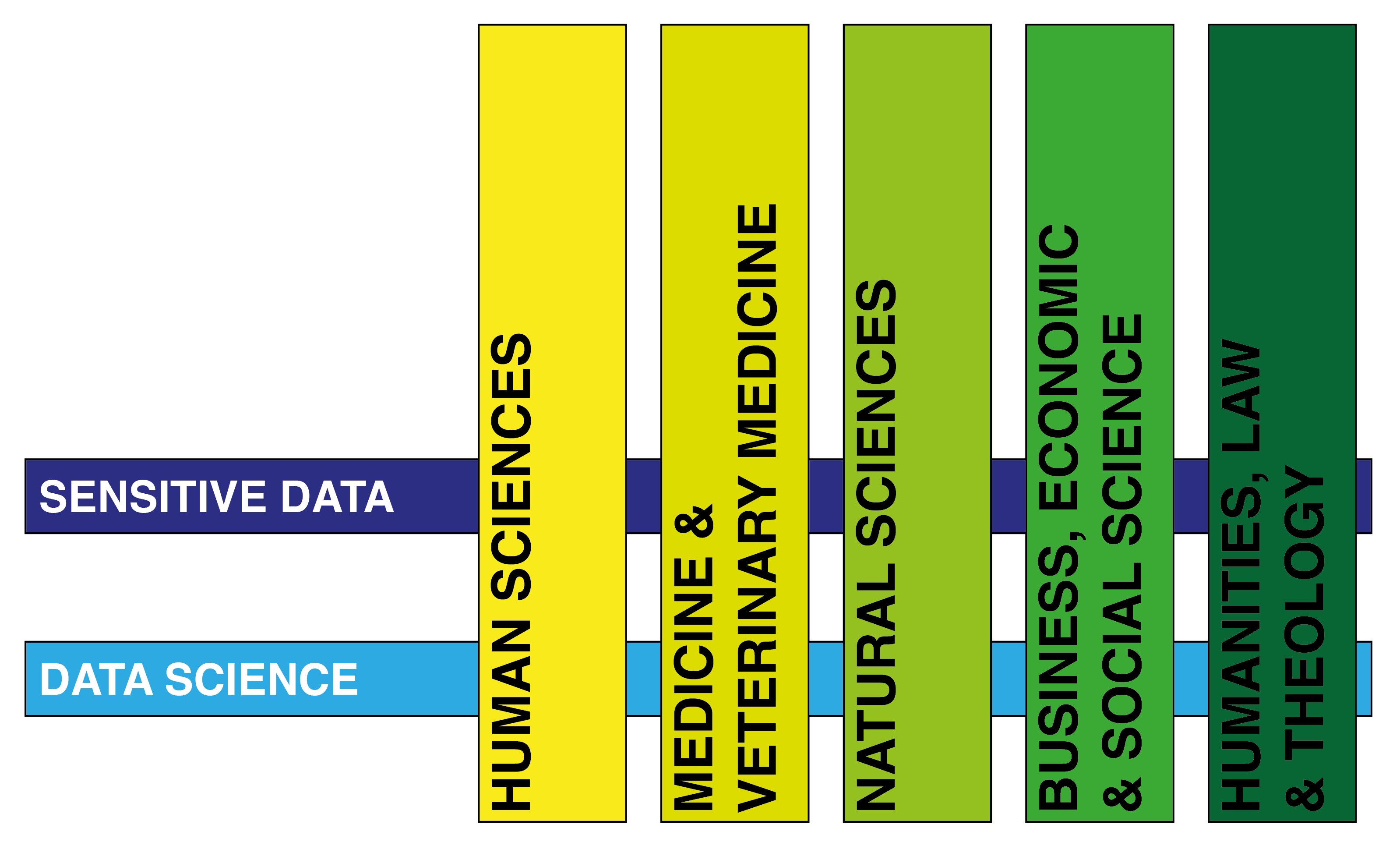
Our Data Stewards are a team of experienced and well-connected research data experts, ready to provide expert support to every faculty and research domain. Specifically, the data stewards are formed by the following scientific staff:
- Data Steward for Medicine and Veterinary Medicine
- Data Steward for Data Science:
- Data Steward for Human Sciences:
- Data Steward for Humanities, Law and Theology:
- Data Steward for Business, Economics and Social Sciences:
- Data Steward for Humanities, Law and Theology:
- Data Steward for Natural Sciences:
The Data Stewards help you to fulfill the funding requirements, edit research proposals regarding Open Science, follow research ethics regarding sensitive data and make your data FAIR available to other researchers and taxpayers. This is done in close cooperation with the data repository BORIS Portal and other support units of the University of Bern.
We offer courses and specifically tailored support for individuals and larger parties. You can find our range of operational courses under Service: Training courses and workshops in Open Science - Bern University Library UB. For other inquiries, please contact us at any time via researchdata@unibe.ch
Glossary
Data Management Plan
A data management plan (DMP) is a structured and document in which the handling of research data in a project is systematically described. It should contain information on how and with which tools (e.g. hardware and software) data is collected, processed, documented, stored, backed up, maintained, archived and, if necessary, published. The DMP also documents the required resources and responsibilities. Ideally, a DMP is drafted during the planning phase of a research project but should be regularly updated and supplemented as the project progresses. The DMP is therefore an instrument for work organization and project planning, but can also help other researchers to interpret and reuse the relevant research data.
Based on: Glossar, Leibniz Universität Hannover (uni-hannover.de)
Data Provenance
Data provenance documents the provenance or origin of research data, and the processes, methods, tools and algorithms used to produce it. Information on the provenance of research data is crucial to ensure transparency/reproducibility of research and thus strengthen its credibility and trust in it. The relevant information can be recorded in readme files or metadata. Provenance information is central to the implementation of the FAIR Data principles.
Based on: eResearch Alliance: Daten-Provenienz
Data Steward
Data stewards are experts in research data management. They support researchers in the sustainable handling of research data. In addition, data stewards act as a link between researchers and (research) software engineers, IT and other infrastructure units. Other tasks include advising, training and raising awareness of good research data management practices.
Based on: https://forschungsdaten.info/praxis-kompakt/glossar/
Data Transfer and Use Agreement (DTUA)
Data Transfer and Use Agreements (DTUA, DUA, or DTA) are contracts that govern the exchange of specific data between two parties. A data agreement establishes the permitted disclosure of a unique data set, allowable uses, and any privacy or security requirements necessary for receiving and handling the data. A data agreement also assigns appropriate responsibilities to the researcher(s) and recipient(s) using the data.
Based on: https://www.purdue.edu/business/sps/contractmgmt/DataTransferUseAgreement.html
Electronic lab notebooks
Electronic lab notebooks (ELN) and laboratory inventory management systems (LIMS) are digital tools that facilitate laboratory work, among other things. ELNs are used to store and record unstructured data, e.g. to organize protocols, notes and data from experiments. LIMS, on the other hand, are intended for structured and repetitive data that follow specific patterns, e.g. when tracking samples from well-defined, repeated and routine tests.
Based on: https://www.scinote.net/blog/eln-vs-lims-how-to-choose/
FAIR Data
The term FAIR (Findable, Accessible, Interoperable and Reusable) Data was first coined in 2016 by the FORCE11 community for practices of sustainable Research Data Management. The main objective of the FAIR principles is the optimal preparation of research data, which should therefore be findable, accessible, interoperable and reusable for both humans and machines.
Based on: FDM-Glossar, Freie Universität Berlin (fu-berlin.de); Wilkinson, Mark, et al. 2016. „The FAIR Guiding Principles for Scientific Data Management and Stewardship“. Scientific Data 3 (1): 160018. https://doi.org/10.1038/sdata.2016.18.
Funder Requirements
Major research funders such as the Swiss National Science Foundation (SNSF), other national research funders or the European Union generally make the release of project funding conditional on the submission of a data management plan and on making research data publicly accessible (Open (Research) Data), provided there are no legal or ethical obstacles.
Based on: https://www.snf.ch/en/dMILj9t4LNk8NwyR/topic/open-research-data
Informed Consent
A declaration of consent (or informed consent) includes informing the participants about what is planned with their data within the scope of a research project, for what purposes the data is to be collected and published, as well as the consent of the participants. Informed consent forms the basis for participation in scientific studies and any subsequent use of the data. It is therefore the basis of research that implements legal provisions and ethical principles.
Licenses for data
A license is a contractually agreed right of use. It allows the rights holder to allow their contractual partner to use a work in various ways (e.g. to copy, save or make it digitally accessible). Standardized Creative Commons licenses or instruments, in particular CC BY and CC0, are generally recommended where copyright claims to research data exist.
Based on https://forschungsdaten.info/praxis-kompakt/glossar, Creative Commons
Long-term archiving
Long-term archiving means securing data and its usability across several generations of hardware, software and file formats.
Based on Universitätsbibliothek Bern, BerDA (German only)
Metadata
Metadata are a highly structured, standardized description of objects (including data). They provide information on content, structure, technical properties, usage rights and other properties in a concise form. Standardized metadata make information findable and usable for machines (e.g. algorithms, search engines). They are therefore crucial to the implementation of the à FAIR Data principles.
Based on: https://en.wikipedia.org/wiki/Metadata
Open (Research) Data
“Open data” in the broad sense refers to all openly accessible and reusable data sets. In a narrower sense, the term is often used synonymously with “open government data” (open administrative data) and in contrast to “open research data” (open research data). Data is open if it is made accessible with as few legal and technical restrictions as possible. If data are released with a highly restrictive license or access barriers (e.g. payment or registration barriers), this can lead to research results not being reproducible, and the subsequent use of the data may be difficult or impossible.
Based on: https://opendatahandbook.org/guide/de/what-is-open-data/
Persistent Identifiers (DOI, ROR et al.)
A Persistent identifier (PID) is a long-lasting digital reference to a digital objects. In contrast to other identifiers such as URLs, PIDs always refer to the object itself. In this way, the identifier does not change, even if the location of the object (usually a website) changes. This ensures permanent traceability. Examples of PIDs are Digital Object Identifiers (DOI), Archival Resource Keys (ARKs), and Handle.
Based on: https://forschungsdaten.info/, CODATA Research Data Management Terminology
Personal Data
According to the Swiss Data Protection Act (DSG), personal data is any information relating to an identified or identifiable natural person. Personal data can be directly identifying information (e.g. name, address, IP address), but also information that can only identify a person in combination with other information (e.g. profession, place of residence).
Based on: Art. 5 DSG Datenschutzgesetz Schweiz | Fact Sheet Datenschutz (Universität Basel)
Personal Data, sensitive
According to the Federal Act on Data Protection (FADP), data relating to health, political or religious beliefs or genetic data, for example, are considered to be sensitive personal data. The protection requirements that apply to this type of data are even stricter compared to non-sensitive personal data.
In Switzerland, different terms are used for this type of data from canton to canton. In the canton of Basel-Stadt, for example, the term “special personal data” is used (IDG §3, para. 4).
Based on: Art. 5 DSG Datenschutzgesetz Schweiz | Kanton Basel-Stadt, Datenschutzgesetz
Preregistration
Preregistration means publishing the plan for a research project before or at the start of a research project. In subject areas such as psychology, the procedure is used to strengthen the method-led approach and increase the quality and transparency of research. The aim is to avoid dubious scientific practice (such as the subsequent adjustment of the research question to the results obtained).
Reproducibility
Research results are reproducible if identical analysis methods are applied to the same data and yield the same results. This can only be achieved if the methods and procedures used are documented correctly and precisely, and all steps performed are documented. Reproducible results enable transparency and traceability. In contrast, replicability means that the results of the replicated study can be confirmed with new data and the same or other methods. However, the definitions of the terms reproducibility and replicability can vary depending on the discipline.
Based on: https://book.the-turing-way.org/reproducible-research/reproducible-research | Plesser HE (2018) Reproducibility vs. Replicability: A Brief History of a Confused Terminology. Front. Neuroinform. 11:76. doi: 10.3389/fninf.2017.00076
Research Data
esearch data are all data that were created or used in the course of research and are considered fundamental for current and potential future knowledge gains in scholarship. In the scholarly community research data are regarded as necessary for the documentation and validation of research results.
A distinction can be made between primary data (data that were collected specifically to answer a research question) and secondary data (data that were collected in a different context and are used in research).
Metadata and documentation of data collection and processing during a research project are essential for the (re)usability of research data (FAIR data). If research data are published under open licenses, it is referred to as open research data.
Research Data Repository
A research data repository is an online platform for the publication of research data. In line with the principles of FAIR Data and Open Research Data, metadata and documentation (e.g. ReadMe file, codebook, protocol) should be entered alongside the research data to make the data easier to find, understand and reuse. To regulate the subsequent use of the data, licenses can be attached to datasets. Access to sensitive data (e.g. personal data) can be restricted and regulated via a data transfer and use agreement (DTUA).
Supplementary Material
Supplementary material (or supplementary data) is material (including data) that cannot be integrated into the main text of a scientific article due to space limitations. This material is not directly necessary to understand the results and conclusions of the article but may still be relevant to the reader for contextualization or further research. It is recommended to publish supplementary material on a publication or research data repository where a DOI or other PID can be assigned to it.
Based on: International Journal of Epidemiology